When using observational data, assignment to treatment groups is nonrandom and causal inferences can be difficult. A common approach to this problem is propensity score weighting, where the propensity score is the probability of assigning a person to a treatment group based on their observable characteristics. This propensity is usually estimated using logistic regression of individual characteristics on a binary variable of whether an individual received treatment or not. Propensity scores are often used to obtain treatment effects adjusted for known confounders by applying an inverse probability of treatment weighting (IPTW) estimator.
a dissertation Xu and so on. (2010) show that using the IPTW method can lead to overestimation of spurious sample sizes and increase the likelihood of Type I errors (ie rejection of the null hypothesis when in fact it is true). The authors claim that robust variance estimators can solve this problem, but only for large sample sizes. Instead, Xu and coauthors propose the use of normalized weights in IPTW as a simple and easy-to-implement strategy. Here’s how it works.
The IPTW method simply examines the difference between treated and untreated groups after applying IPTW weights. How often to get someone in for treatment:
Where n1 is the number of people treated no is the total sample size.Let z=1 if the person is processed in the data and z=0 if the person is not treated. Suppose each person has a patient feature vector, x, which can affect the likelihood of receiving treatment. Then calculate the treatment probability as:

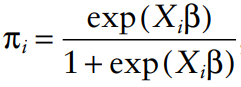
Under standard IPTW, the weights used are:
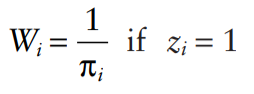
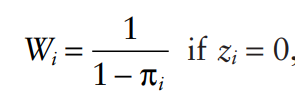
Xu and coauthors created a simulation to show that the Type 1 error was too high—typically 15% to 40%. To correct this, normalized weights (SW) can be used as follows:
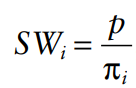
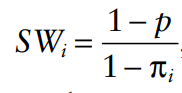
The former is used in the treated population (ie z=1), and the latter is used in the untreated population (z=0). The authors show that, under normalized weights, Type 1 errors occur at about the expected 5% rate. In fact, the authors also show that standardized weights generally outperform robust variance estimators in estimating main effects.
For more information, you can read the full text here.